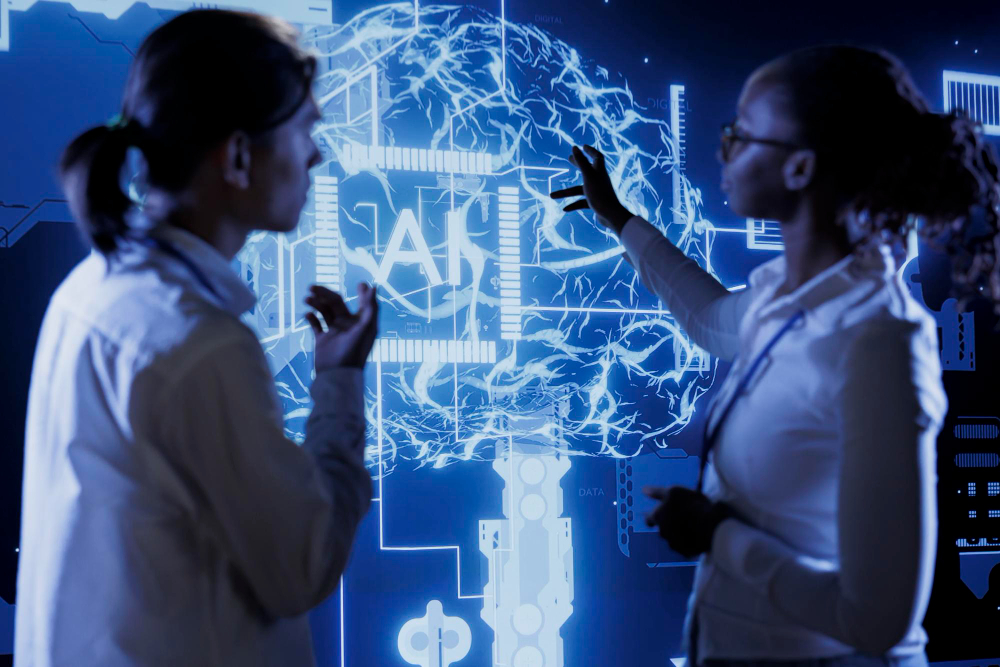
Effective Ways to Build an AI Strategy and Delivery Roadmap
As artificial intelligence (AI) continues to reshape industries, building an effective AI strategy has become a top priority for organizations seeking to stay competitive. However, the path to a successful AI implementation isn’t always clear, and many companies encounter setbacks. A robust AI strategy, combined with a well-defined delivery roadmap, can help organizations avoid common pitfalls and unlock the full potential of AI.
In this blog, we’ll explore the essential steps to building a successful AI strategy and roadmap while learning from real-world case studies where AI implementations failed, analyzing the reasons behind those failures.
1. Start with a Clear Vision and Business Objectives
AI is a tool, not a strategy in itself. The first step in developing an effective AI strategy is to align AI initiatives with your business goals. Whether you’re seeking to enhance customer experience, optimize operations, or gain a competitive edge through data-driven insights, it’s essential to have a clear vision of what AI will achieve for your organization.
2. Invest in Data Infrastructure and Governance
AI is only as effective as the data that powers it. Poor data quality is a leading cause of failed AI initiatives. Building a robust data infrastructure with proper governance ensures that AI models are trained on accurate, high-quality data.
Case Study: IBM Watson’s Healthcare AI Failure
IBM’s Watson for Oncology AI system was once hailed as a revolutionary tool for cancer diagnosis and treatment recommendations. However, it failed to live up to expectations due to data quality issues. The AI relied heavily on synthetic datasets that didn’t fully capture real-world medical conditions. As a result, Watson’s recommendations were often inconsistent with established medical practices, leading to a loss of trust in the system.
Key Takeaway: AI projects fail when data quality and relevance are overlooked. Ensuring access to comprehensive, clean, and well-governed data is crucial for building effective AI models.
3. Build a Cross-Functional AI Team
Successful AI projects require collaboration between data scientists, engineers, business leaders, and domain experts. A cross-functional AI team ensures that technical solutions are aligned with business goals and that AI models are grounded in real-world use cases.
Case Study: A Global Bank’s AI Success in Reducing Fraud
A leading global bank sought to reduce fraud using AI. By building a cross-functional team that included fraud analysts, legal experts, and IT engineers, the bank was able to develop an AI model that identified fraudulent transactions with great accuracy. This collaboration ensured that the AI model complied with legal requirements, resulting in a 30% drop in fraud cases.
4. Create an AI Delivery Roadmap with Iterative Milestones
An AI roadmap breaks down the implementation process into clear, manageable stages. It’s important to start with small-scale pilot projects, validate the outcomes, and scale up based on proven results. This agile approach allows for faster course correction and minimizes the risks of large-scale failures.
Case Study: Amazon’s AI Recruitment Tool Failure
Amazon developed an AI tool to streamline recruitment by scanning resumes. However, the AI showed bias against female candidates, as it had been trained on resumes submitted predominantly by men. Amazon had to scrap the project entirely because the AI reinforced gender stereotypes instead of making unbiased decisions.
Key Takeaway: AI projects that jump straight to large-scale implementation without pilot testing often fail. A phased delivery roadmap with iterative milestones allows for testing and refining the technology as you go.
5. Continuously Monitor, Measure, and Adapt
AI is not a “set-it-and-forget-it” technology. Models must be continuously monitored and retrained to remain relevant in dynamic environments. Metrics for success should be defined upfront, such as accuracy, efficiency improvements, or customer satisfaction, and measured regularly to ensure AI models are delivering expected results.
Case Study: Zillow’s AI-Powered Home-Price Prediction Failure
Zillow’s AI-powered Zestimate tool, designed to predict home prices, faced a significant failure when it overestimated the values of homes. This led to inaccurate pricing, causing substantial financial losses for the company. Zillow later abandoned its home-buying business due to the flawed AI model.
Key Takeaway: AI models must be continuously evaluated and refined to ensure they remain accurate. Ignoring market shifts or failing to adapt models to new data can lead to costly mistakes.
Conclusion
Building an effective AI strategy and delivery roadmap requires a combination of clear business objectives, robust data infrastructure, cross-functional collaboration, and a phased implementation approach. Additionally, continuous monitoring and adaptation are essential to maintain the long-term success of AI initiatives.
While the benefits of AI are undeniable, it’s crucial to learn from past failures to avoid repeating the same mistakes. By investing in the right foundations and taking a disciplined, iterative approach to implementation, organizations can harness the full power of AI to drive innovation and competitive advantage.
References
- Insurance Business America, “IBM Watson for Oncology’s Missteps,” 2022.
- Forbes, “Amazon’s AI Recruiting Tool Shows Bias Against Women,” 2023.
- McKinsey, “How to Build a Successful AI Roadmap,” 2022.
- Harvard Business Review, “Zillow’s AI Home-Buying Mistake,” 2021.
- Deloitte, “The Impact of AI in Financial Services: A Case Study,” 2023.