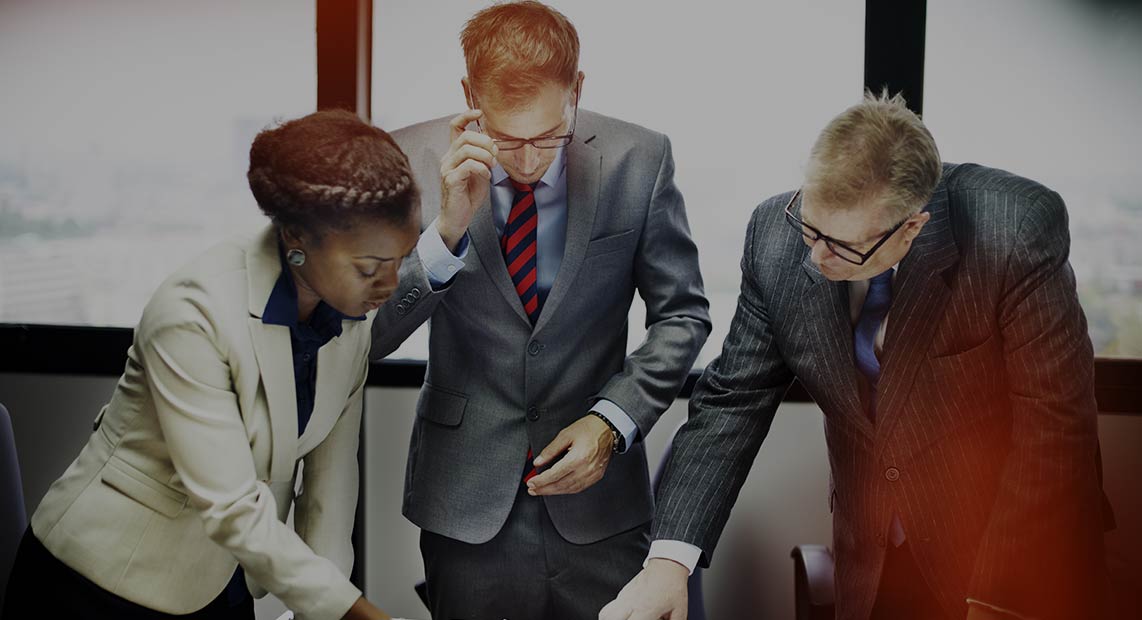
The Importance of a Strong Data Strategy
Data Governance, Quality, and Business Alignment
In today’s data-driven world, businesses are increasingly reliant on high-quality data to make informed decisions, drive innovation, and maintain a competitive edge. However, without a robust data strategy, organizations face significant challenges, including poor data governance and compromised data quality. A strong data strategy not only ensures that data is accurate, reliable, and accessible but also aligns data initiatives with business goals, enabling organizations to fully leverage their data assets.
In this blog, we’ll explore the importance of a strong data strategy, showcase the risks associated with poor data quality, and examine how businesses play a crucial role in aligning data goals with their broader objectives.
The Need for a Strong Data Strategy
A strong data strategy provides the foundation for how data is collected, managed, and used across an organization. It involves setting a clear vision for how data will support business goals, and it outlines the processes and technologies needed to achieve this.
Key components of a data strategy include:
- Data Governance: Ensures that data is properly managed and maintained, defining who is responsible for data quality, security, and compliance.
- Data Quality: Ensures the accuracy, completeness, and reliability of data, which is critical for decision-making and operational efficiency.
- Business Alignment: Ensures that the data strategy aligns with the company’s overall goals, helping to solve real-world problems and uncover new opportunities.
A comprehensive data strategy provides the blueprint for how data initiatives are prioritized, governed, and executed, ensuring that data becomes a strategic asset rather than a liability.
The Fallout of Poor Data Quality
Case Study: JP Morgan’s $6 Billion Trading Loss
One of the most well-known examples of the consequences of poor data quality is the 2012 JP Morgan trading scandal, also known as the “London Whale” incident. The company’s risk model failed due to incorrect data inputs, resulting in a massive $6 billion loss. Flawed data, compounded by a lack of proper data governance, led to incorrect risk assessments and poor decision-making.
Key Takeaway: Without strong data governance and quality controls, even a financial giant like JP Morgan can suffer significant losses. Ensuring data accuracy and reliability is crucial in high-stakes environments.
Case Study: A Telecom Company’s Customer Data Crisis
A leading telecom provider faced challenges in managing its customer data, leading to inaccurate billing and delayed service activation. A lack of data governance and poor data quality management caused discrepancies in customer information across different systems. As a result, the company faced significant customer churn and a damaged reputation.
Key Takeaway: When data quality is compromised, customer satisfaction can plummet. Data governance and quality checks are essential for businesses that rely heavily on accurate customer data.
The Role of Data Governance and Quality
Data governance refers to the framework that ensures data is managed properly, in compliance with regulations, and available for use across the organization. Without a solid data governance framework, organizations are vulnerable to inefficiencies, errors, and regulatory risks.
Why Data Quality Matters
Poor data quality leads to erroneous insights, which can severely impact decision-making. A Gartner study found that poor data quality costs organizations an average of $15 million per year in losses, and those with low data quality are more likely to experience failed projects or incorrect business decisions.
Key factors affected by poor data quality include:
- Decision-Making: If data is inaccurate or incomplete, business leaders cannot make informed decisions, leading to missed opportunities or costly mistakes.
- Operational Efficiency: Low-quality data can disrupt operations, causing delays and inefficiencies in processes such as customer service, marketing, and supply chain management.
- Compliance Risks: In industries like finance and healthcare, poor data quality can lead to non-compliance with regulations, resulting in legal penalties or reputational damage.
The Role of Business in Ensuring Data Strategy Success
A successful data strategy is not solely the responsibility of the IT or data teams. Business leaders play a crucial role in defining the vision for data and ensuring it aligns with the organization’s overall goals. Understanding the specific problems they need to solve and clearly defining expectations is essential to building an effective data infrastructure.
- Identifying Data Gaps and Needs: Business leaders must work closely with data teams to identify existing data gaps. This involves understanding where data is missing, inconsistent, or inaccurate and how it affects business outcomes. Defining what data is needed and the role it plays in achieving business goals ensures that data initiatives are aligned with broader organizational objectives.
- Establishing Clear Data Objectives : Organizations must have a clear vision of what they want their data to accomplish. Whether it’s improving customer experiences, optimizing operational efficiencies, or driving innovation, businesses must establish specific objectives for how they plan to leverage data to achieve these outcomes
- Aligning Data Strategy with Business Problems : Businesses need to understand the problems they are trying to solve and ensure that their data infrastructure can address those challenges. This requires a strong alignment between business goals and data strategies. For example, if a company aims to improve customer retention, their data strategy should focus on analyzing customer behavior and trends through reliable, high-quality data..
Aligning Data Strategy with Business Problems
Ford Motor Company realized that poor data quality and siloed data systems were impeding its ability to analyze customer preferences and innovate effectively. By implementing a comprehensive data governance framework and aligning its data strategy with business goals, Ford was able to streamline its operations and significantly improve customer insights. The company now uses high-quality data to drive innovation in areas such as vehicle design and customer experiences, leading to higher customer satisfaction and operational efficiency.
Key Takeaway: When businesses take an active role in data strategy development and data quality improvement, they are better positioned to solve business problems and innovate.
Building a Strong Data Infrastructure
The foundation of any successful data strategy is a strong data infrastructure. This includes the technologies, processes, and policies required to ensure that data is collected, stored, and accessed efficiently. Without the right infrastructure in place, even the best data strategy will fail.
Key elements of a robust data infrastructure include:
- Cloud Platforms: These enable organizations to store and manage large volumes of data efficiently and securely.
- Data Lakes and Warehouses: Centralized repositories that allow for easy access and analysis of data from multiple sources.
- Data Governance Tools: Platforms that provide data governance capabilities, ensuring data quality, compliance, and security.
Conclusion
A strong data strategy, built on the pillars of data governance, quality, and business alignment, is essential for any organization looking to thrive in today’s digital world. The risks of poor data quality are clear—from financial losses to damaged reputations. By investing in data governance and infrastructure, businesses can unlock the full potential of their data, drive innovation, and maintain a competitive edge.
Business leaders must take an active role in defining data goals, identifying gaps, and ensuring their data strategy aligns with the organization’s broader objectives. With the right vision, infrastructure, and quality controls in place, organizations can leverage their data to solve real-world problems and capitalize on new opportunities.
References
- Gartner, “The High Cost of Poor Data Quality,” 2021.
- Financial Times, “The $6 Billion ‘London Whale’ Trading Loss,” 2012.
- Harvard Business Review, “How Ford Aligned Its Data Strategy to Business Goals,” 2020.
- Forbes, “Customer Data and Quality Issues in Telecom: A Case Study,” 2019.
- IBM, “Data Governance and the Future of Data-Driven Decision Making,” 2023